Improved assimilation algorithm
SEEDS develops an advanced data assimilation algorithm (4DEnVar) to prepare the way for better exploitation of the hourly data from Sentinel 4 and improve air quality forecasts in the CAMS operational system.
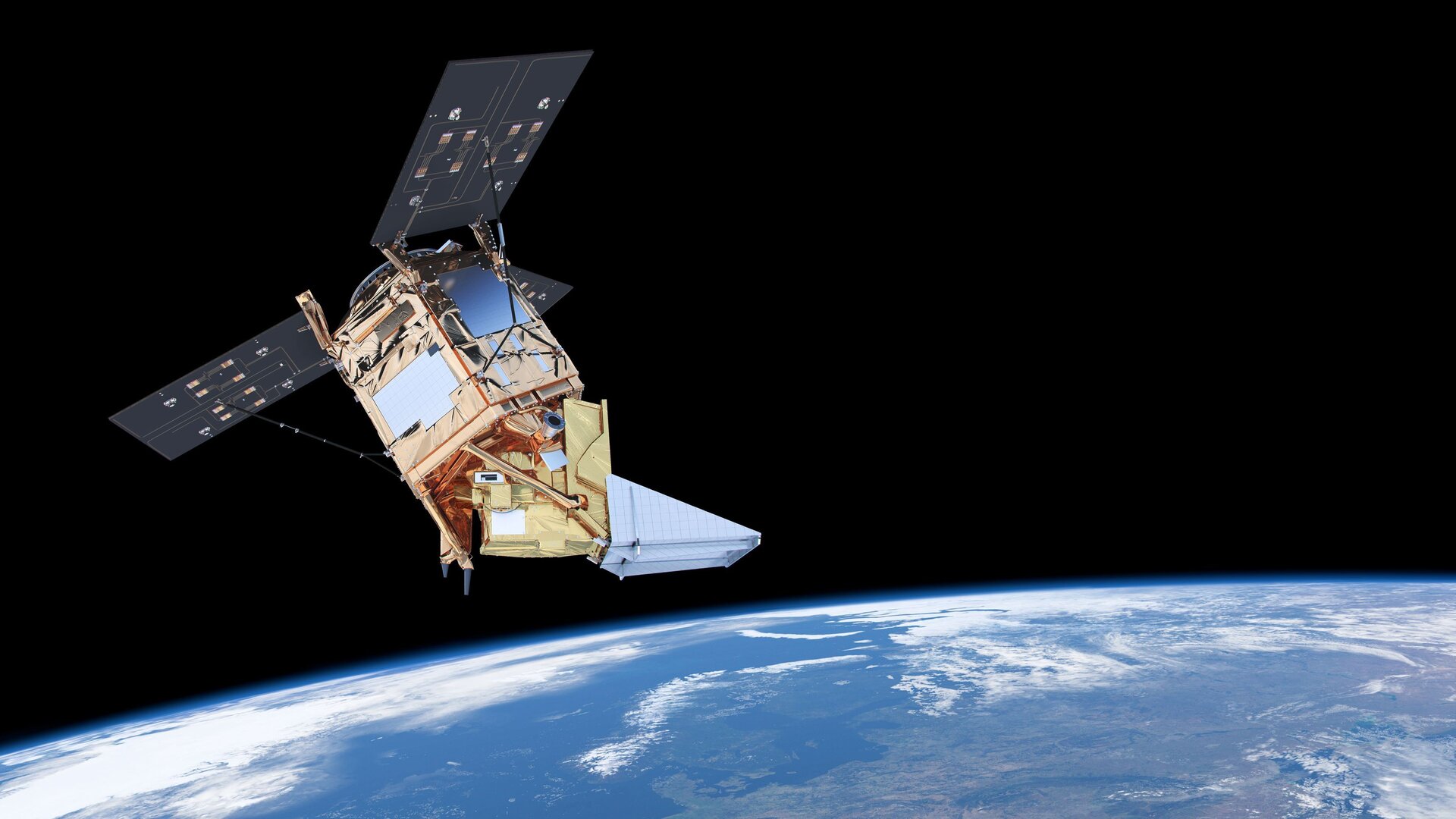
SEEDS’ new data assimilation algorithm optimally integrates different models, by addressing the majority of errors causing uncertainty in air quality predictions.
- SEEDS methodology for advanced products data assimilation
- Open-source code with the 4DEnVAr algorithm for use by a wide range of researchers and scientific experts
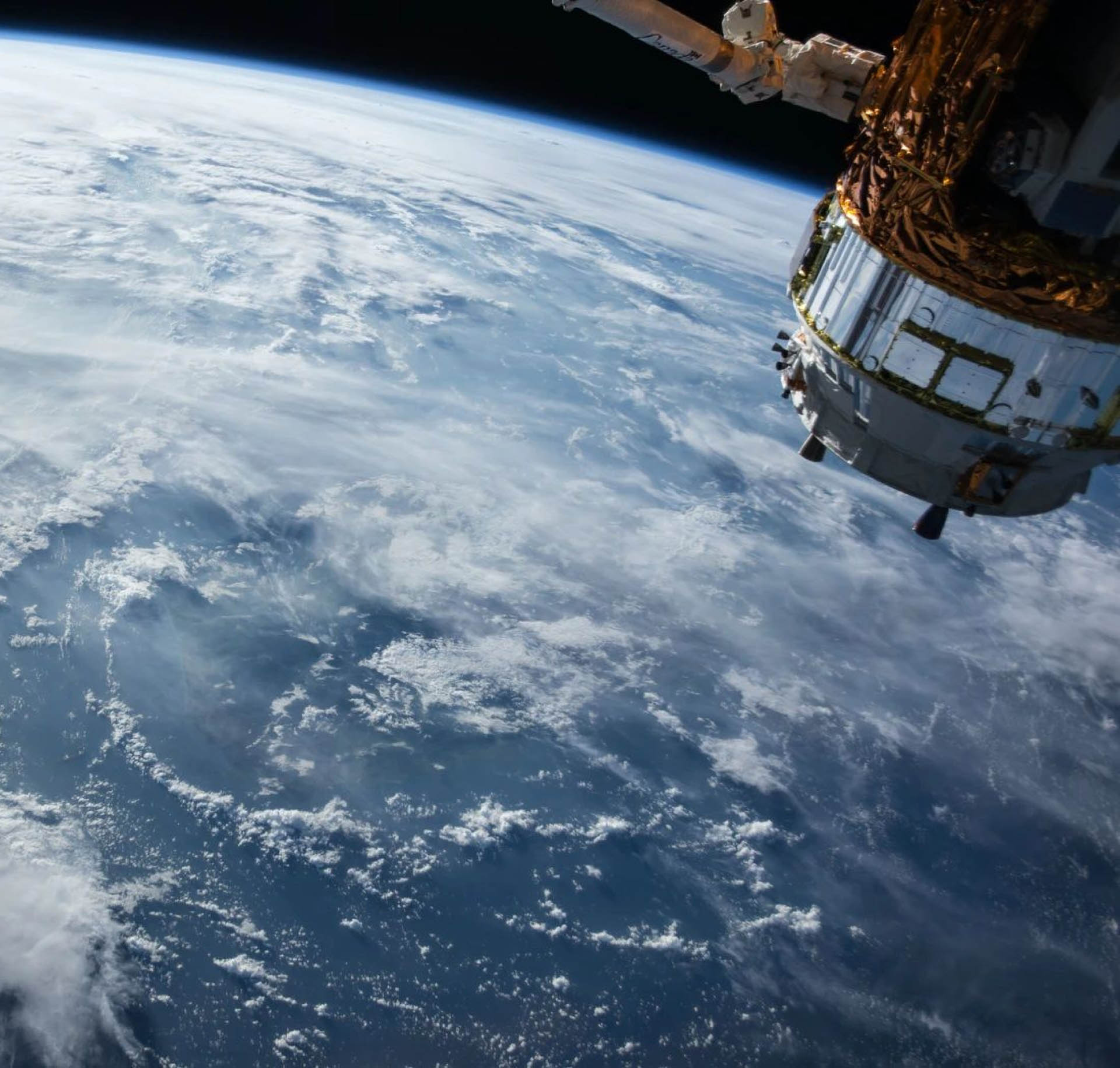
The 4DEnVar is a hybrid algorithm that includes information from probabilistic forecasts with variational (4D-Var) and ensemble-based approaches (EnKF) to make the best out of these two different Data Assimilation (DA) schemes.
The new 4DEnVAr data assimilation algorithm combines the capacity to assimilate highly resolved temporal variability of observations and to account for the presence of multiple competing error terms including those from emission inversion models. It is able to account for various types of model errors deriving from different DA schemes, which are responsible for a significant part of the uncertainty in air quality predictions. It also allows to reduce analyses and forecast biases that are not related to emissions/deposition or derive from emissions/deposition not well constrained.
The SEEDS new assimilation algorithm is made to address and reduce model errors for next-day air-quality forecasts. It works both based on an ensemble of air-quality forecasts provided by a single perturbed model or based on a collection of different models (CAMS) to allow for extended use by the research community. It takes advantage of frequent (hourly) observations (in-situ, Sentinel4) allowing the use of daily information instead of one single observation per hour and station.